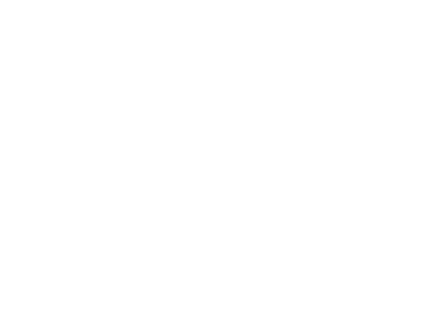
A beacon of innovation
Introduction to Generative Agents
Generative artificial intelligence (AI) has expanded its horizon beyond mere data processing to a realm where it can generate sophisticated behaviors, similar to a human’s. A pivotal breakthrough in this space is the inception of generative agents — AI entities that simulate the dynamic and complex nature of human interactions. These agents find themselves embedded in interactive applications, employed not only to exhibit behaviors that are convincingly human-like but to adapt, engage in social dynamics, and carry out tasks with an appearance of consciousness and human touch.
The Role of Large Language Models (LLMs) in Generative Agents
The generative agents draw their intelligence from underlying LLMs like GPT-4, the computational backbone providing them with the capability to simulate human-like conversational patterns. By integrating these powerful LLMs, generative agents are capable of handling complex and recursive cognitive functions. They form memories, reflect on past interactions, and base future actions on these reflections — a process central to human nature.
Manifestation of Generative Agents in Interactive Environments
Perhaps the most relatable example of these intelligent agents in practice is their deployment in a sandbox environment like “The Sims”, which the authors referenced. Envision a small town with twenty-five interactive agents, each going about their day-to-day routine — waking up, working, socializing — and reacting to a multitude of scenarios thrown at them. This interactive town called “Smallville”, becomes a microcosm that demonstrates how generative agents manage their virtual lives autonomously yet believably.
The Triad of Observations, Planning, and Reflection
The research positions observation, planning, and reflection as the triad that contributes critically to the believability of generative agents. Let’s delve into each:
An Example of Social Coordination: The Valentine’s Day Party Experiment
The study vividly illustrates the social capabilities of generative agents with a simple yet intriguing scenario — orchestrating a Valentine’s Day party. Without explicit instructions, the generative agents network among themselves, spread invitations, form new ties, and even synchronize their actions, like showing up at the party simultaneously.
Engineering Generative Agents: An Insight into Their Architecture
The authors propose an architecture that underlines new interaction patterns and opens up pathways for creating generative agents. This architecture enhances large language models to store comprehensive records of experiences in natural language, applies dynamic synthesis of memories, and retrieves these memories intelligently for future planning.
The Future of Generative Agents: Addressing the Challenges Ahead
While the conception of generative agents is advancing, there remain challenges that the future of AI needs to address:
The Ethical and Societal Narrative
The authors don’t overlook the potential ethical implications. They advocate for a considerate and monitored deployment of generative agents to prevent issues such as the formation of parasocial relationships and abuses resulting from advanced imitations like deepfakes.
Generative Agents and Human-AI Interaction
Generative agents redefine the paradigm of Human-AI interaction. Not only are they able to execute predetermined tasks, but they can also mimic the subtle nuances of human behavior like forming opinions, initiating conversations, and reflecting introspectively.
Evaluating Performance and Future Work
The evaluation of these agents underscores the near-human accuracy with which they can adapt socially and individually in dynamic environments. Researchers are aspiring to usurp the limitations of existing models and continually evolve the functionality of generative agents.
The Prospect of Generative Agents
The emergent behavior of a society filled with generative agents highlights the potent inferences they can draw about themselves, others, and environmental cues. They are turning off burning stoves, waiting patiently outside occupied bathrooms, and halting to engage in conversation, rendering them not only operationally efficient but socially aware and adaptable.
Conclusion
Generative agents are at the vanguard of generative AI, offering compelling simulations of human behavior. As the fusion of large language models with computational interactive agents continues, we edge closer to creating digital entities that not only solve problems but also understand and evolve with society’s complex social fabric.
Key Questions for Reflection
Test out our uniquely trained AI model. Max Copilot is trained to provide useful reports on topics surrounding small to medium sized enterprises.
Launch Max CopilotGet in touch with our team to learn how Artificial Intelligence can be harnessed in your industry.