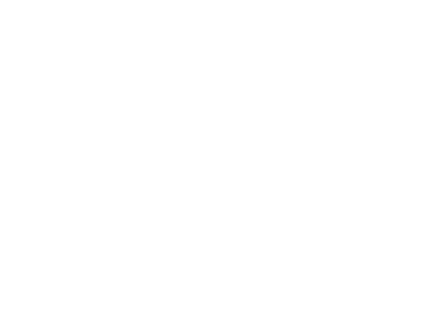
A beacon of innovation
Comprehensive Framework for Ethical Principles in Generative Artificial Intelligence
1. Executive Summary
The advent of Generative Artificial Intelligence (AI) has been accompanied by remarkable capabilities and innovations that promise to reshape industries and human experiences. However, as with any disruptive technology, it has also surfaced ethical complexities that demand careful consideration and robust frameworks to guarantee its positive influence on society. This document outlines comprehensive ethical principles tailored specifically for generative AI systems, synthesizing interdisciplinary research, industry practices, and sociotechnical insights.
2. Introduction
Generative AI refers to a class of AI that specializes in creating content, ranging from text to images, and engaging in interactions that require a certain degree of creativity or simulation of human-like thought processes. As these systems blur the lines between human and machine-generated content, establishing ethical principles that guide the development, deployment, and governance of generative AI is critical.
3. Ethical Foundations and Societal Impact
Human-Centric Values:
Ethical AI must prioritize human rights and well-being. It must be designed with a human-in-the-loop approach that facilitates augmentation, not replacement, and ensures accountability and comprehensiveness.
Equity and Fairness:
Generative AI must mitigate biases that perpetuate discrimination and inequality. This involves the creation of diverse and inclusive datasets, regularly auditing models for bias, and the implementation of ethical use cases.
Transparency and Explainability:
Stakeholders must understand how generative AI systems operate. Transparent practices and clear communication about system capabilities and limitations are essential for building trust.
Privacy and Data Protection:
Generative AI should uphold the highest standards of data privacy and security. This involves adherence to data protection regulations and the safeguarding of personal and sensitive information.
Safety and Security:
Systems must be robust against adversarial attacks and misuse. Continuous risk assessment and mitigation strategies must be in place to protect against potential harms.
Environmental Sustainability:
Given the significant energy demands of training large models, generative AI must strive for sustainable practices, reducing carbon footprint and promoting green AI initiatives.
4. Specific Principles for Generative AI Systems
Avoidance of Unfair Bias:
Algorithmic fairness must be a cornerstone to prevent the propagation of stereotypical, misleading, or harmful content. Measures such as fairness indicators and impact assessments should be routine.
Societal and Environmental Wellbeing:
The deployment of generative AI should contribute positively to society, respecting human values, promoting social welfare, and advocating for sustainable approaches.
Governance and Oversight:
Multistakeholder governance structures must be established to oversee ethical generative AI development, inclusive of policymakers, technologists, social scientists, ethicists, and civil society representatives.
Accountability:
Clear lines of responsibility must exist for decisions made by or with the help of generative AI. Companies and operators should be accountable for the outputs and impacts of their AI systems.
Continuous Ethics Education:
Stakeholders, including developers and users, must be provided with ongoing ethics training to recognize potential ethical issues and take corrective actions.
5. Technical Foundations and Design Ethics
Transparency, Accuracy, and Fairness
Generative AI applications should be designed with transparency in mind, providing stakeholders with clarity on how AI systems operate and how their outputs are determined. Accuracy is paramount, necessitating the establishment of stringent measures to ensure AI-generated content is reliable and trustworthy. Additionally, fairness must be embedded into the design process to avoid perpetuating biases, ensuring equitable outcomes across diverse user groups.
Data Privacy and Security
The lifecycle of AI data, from sourcing and processing to storage, should adhere to robust privacy and security standards. Principles dictated by regulatory frameworks such as the GDPR inform this stance, prioritizing user consent and data minimization. Secure protocols must be established to safeguard the data against breaches and unauthorized access.
Robustness and Reliability
Robust AI algorithms are developed through rigorous testing and continuous improvement, designed to withstand adversarial attacks and unpredictable scenarios. Measures to counter biases and prevent unintended consequences stand at the center of creating reliable generative AI solutions, ensuring these systems perform as intended.
6. Planning and Implementation Ethics
Stakeholder Engagement and Risk Assessment
Stakeholder engagement is pivotal in planning ethical AI, requiring the involvement of individuals who will be affected by the AI systems in their conceptualization and design. Comprehensive risk assessments should be undertaken to identify potential drawbacks and formulate appropriate mitigation strategies.
Interdisciplinary Collaboration
The fabric of ethical AI development is interwoven with threads from varied disciplines. Engaging engineers, ethicists, domain experts, and a strong collaboration between these diverse vanguards energizes the design and deployment of responsible AI. This alliance between disciplines fosters innovations that respect ethical boundaries while pushing the frontiers of what AI can accomplish.
7. Negative Consequences and Mitigation
Addressing Negative Outcomes
The potential for negative consequences, such as job displacement, generation of misinformation, and societal manipulation, necessitates proactive measures. Strategies for mitigation include implementing ethical design practices, regulatory compliance, and continuous monitoring to detect and address harm.
Ethical Audits
Regular ethical audits of generative AI applications can help identify risks and non-compliance issues, providing the necessary insights to enhance ethical standards.
8. Ethical AI Governance
Policies, Standards, and Guidelines
Governance frameworks serve as the blueprints for ethical AI, enumerating standards and guiding policies. These frameworks should embed within organizational structures, ensuring adherence that is regulated as much by internal compasses as by governmental edicts. Ethical AI governance forms the skeletal structure that upholds ethical AI practice.
International Cooperation
The ethos of AI ethics is not confined by borders but rather is nourished by global cooperation and shared standards. These universal norms are critical in manifesting AI systems that respect diverse cultural prerogatives, allowing for technological innovations to be genuinely global in their reach and impact.
9. Regulatory and Policy Considerations
Alignment with Global Standards:
Generative AI must align with established international frameworks, such as GDPR for privacy, to ensure consistent ethical applications across borders.
Adaptive Legislation:
Policymaking should be adaptive and informed by ongoing research and experiences, evolving alongside the advancements in AI technology.
Ecosystem Collaboration:
Policymakers should collaborate with academia, industry, and civil society to create an informed ecosystem guiding generative AI legislation.
Ethical Certification:
Implementing a certification system for ethical AI could encourage organizations to adhere to high standards and offer assurance to users and regulators.
10. Challenges and Future Directions
Evolving Technologies:
As generative AI evolves, so must the ethical frameworks that guide it. This demands ongoing and iterative dialogue among stakeholders.
Global Consensus:
Developing a global consensus on ethical AI norms remains challenging due to cultural and political differences, but is essential for widespread ethical integration.
Measurement of Impact:
Assessing the societal impact of generative AI is complex. Effective tools and methodologies for measuring and evaluating such impact are necessary.
11. Future Insights and Evolution of AI Ethics
Anticipating Technological Trends
Predicting future trends in AI technology equips policymakers and developers to preemptively address evolving ethical considerations. As generative AI systems grow more sophisticated, ethical guidelines must similarly advance to remain relevant and effective.
Future Trends in AI Ethics
The highway of AI progress is one of constant change, with new generations of generative capabilities on the horizon. Ethical frameworks must be agile, capable of adapting to new challenges, and insist on a forward-looking stance that factors in the rapid pace of technological evolution to defend human values.
AI-Generated Content Guides
The creation of AI-generated content, while burgeoning in ubiquity, requires meticulous guidance. The production of such content must be underpinned by principles of clarity, contextuality, and relevance to ensure that the AI serves as a credible contributor to the ecosystem of information and creative expression.
AI Memory Usage in Conversational Agents
Conversational agents represent a unique facet of AI, serving as interspersing interfaces between humans and machines. The ethical use of memory in these agents reflects the need to balance data retention for coherence and an unyielding respect for privacy.
12. Ensuring Ethical Use
Application in Varied Contexts
Ensuring ethical application requires tailored solutions that factor in the nuances of different contexts where generative AI operates. It is vital to create frameworks flexible enough to be adapted to a range of environments while maintaining their core ethical standards.
13. Conclusion
The creation of ethical generative AI systems is pivotal not just for maintaining public trust but for fostering a technologically advanced society that prioritizes humanity’s collective well-being. The proposed comprehensive framework offers a multi-faceted approach to address the profound challenges of generative AI and pave the way for responsible innovation. It is a living document, subject to refinement as the landscape of generative AI and its societal implications continue to evolve.
References
Test out our uniquely trained AI model. Max Copilot is trained to provide useful reports on topics surrounding small to medium sized enterprises.
Launch Max CopilotGet in touch with our team to learn how Artificial Intelligence can be harnessed in your industry.